Forschungsgruppe Statistische Methoden der Kausalen Inferenz
Die Forschungsgruppe befasst sich mit statistischen Methoden, um aus Beobachtungsstudien möglichst zuverlässige Schlüsse darüber zu ziehen, welches die zu erwartenden Konsequenzen von konkreten (manchmal auch hypothetischen) Interventionen sein würden. Solche Erkenntnisse tragen wesentlich zur Planung zukünftiger Interventionsstudien bei und sind letztlich für die Entscheidungsfindung im öffentlichen Gesundheitswesen von entscheidender Bedeutung, insbesondere in Hinblick auf präventive Maßnahmen und Strategien. Die statistische Herausforderung liegt darin, dass Schwachstellen der verfügbaren Daten, z.B. fehlende oder imperfekte Randomisierung, nicht-zufällige Selektion oder Drop-out etc., so gut wie möglich entgegengewirkt wird bzw. diese adäquat berücksichtigt und z.B. mitmodelliert werden.
Der Schwerpunkt dieser Forschungsgruppe liegt darauf, die theoretischen und praktischen Eigenschaften relevanter Methoden näher zu analysieren und zu vergleichen, aber vor allem auch solche Methoden zu entwickeln und bereitzustellen, die für konkrete Studienauswertungen im BIPS wesentlich sind. Eine Grundvoraussetzung dazu ist ein detailliertes Verständnis und Durchleuchten der jeweiligen Annahmen, sodass diese empirisch und durch Vorwissen gerechtfertigt werden können.
Die Forschungsinteressen der Forschungsgruppe sind:
- kausale Modellierung und Methoden für zeitabhängige Daten, vor allem veränderliche Expositionen
- Target Trial Emulierung (siehe AG GeTTCausal)
- Kausale Modellselektion mit zeitabhängigen Daten
- Machinelles Lernen für kausale Inferenz
- Modellierung und Methoden der kausalen Pfadanalyse
- Instrumentelle Variablen, insbesondere „Mendelian Randomisation“
- Bias Modellierung und Analyse
Ausgewählte Veröffentlichungen
- Bang CW, Didelez V. Do we become wiser with time? On causal equivalence with tiered background knowledge. In: Evans R, Shpitser I, Herausgeber. Proceedings of the thirty-ninth conference on uncertainty in artificial intelligence (UAI 2023), Pittsburgh, USA. Volume 216. 2023. S. 119-129.
https://proceedings.mlr.press/v216/bang23a - Braitmaier M, Didelez V. Emulierung von Target Trials mit Real World Daten - Ein allgemeines Prinzip, um den Herausforderungen von Beobachtungsdaten zu begegnen. Prävention und Gesundheitsförderung. 2025; (Epub 2022 Jul 29).
https://doi.org/10.1007/s11553-022-00967-9 - Evans R, Didelez V. Parameterizing and simulating from causal models. Journal of the Royal Statistical Society. Series B (Statistical Methodology). 2024;86(3):535-568.
https://doi.org/10.1093/jrsssb/qkad058 - Foraita R, Witte J, Börnhorst C, Gwozdz W, Pala V, Lissner L, Lauria F, Reisch LA, Molnár D, De Henauw S, Moreno LA, Veidebaum T, Tornaritis M, Pigeot I, Didelez V. A longitudinal causal graph analysis investigating modifiable risk factors and obesity in a European cohort of children and adolescents. Scientific Reports. 2024;14:6822.
https://doi.org/10.1038/s41598-024-56721-y - Do S, Didelez V, Börnhorst C, Coumans J, Reisch LA, Danner U, Russo P, Veidebaum T, Tornaritis M, Molnár D, Hunsberger M, De Henauw S, Moreno LA, Ahrens W, Hebestreit A, on behalf of the IDEFICS and I.Family consortia. The role of psychosocial well-being and emotion-driven impulsiveness in food choices of European adolescents. International Journal of Behavioral Nutrition and Physical Activity. 2024;21:1.
https://doi.org/10.1186/s12966-023-01551-w - Schreuder A, Börnhorst C, Wolters M, Veidebaum T, Tornaritis M, Sina E, Russo P, Moreno LA, Molnár D, Lissner L, De Henauw S, Ahrens W, Vrijkotte T, on behalf of the GrowH! consortium. Population trajectories and age-dependent associations of obesity risk factors with body mass index from childhood to adolescence across European regions: A two-cohort study. Pediatric Obesity. 2024;19(2):e13088.
https://doi.org/10.1111/ijpo.13088 - Börnhorst C, Ahrens W, De Henauw S, Hunsberger M, Molnár D, Moreno LA, Russo P, Schreuder A, Sina E, Tornaritis M, Vandevijvere S, Veidebaum T, Vrijkotte T, Wijnant K, Wolters M, on behalf of the GrowH! consortium. Age-specific quantification of overweight/obesity risk factors from infancy to adolescence and differences by educational level of parents. International Journal of Public Health. 2023;68:1605798.
https://doi.org/10.3389/ijph.2023.1605798 - Koelman L, Herpich C, Norman K, Jannasch F, Börnhorst C, Schulze MB, Aleksandrova K. Adherence to healthy and sustainable dietary patterns and long-term chronic inflammation: Data from the EPIC-Potsdam cohort. The Journal of Nutrition, Health & Aging. 2023;27(11):1109-1117.
https://doi.org/10.1007/s12603-023-2010-1 - Börnhorst C, Pigeot I, De Henauw S, Formisano A, Lissner L, Molnár D, Moreno LA, Tornaritis M, Veidebaum T, Vrijkotte T, Didelez V, Wolters M, on behalf of the GrowH! consortium. The effects of hypothetical behavioral interventions on the 13-year incidence of overweight/obesity in children and adolescents. International Journal of Behavioral Nutrition and Physical Activity. 2023;20:100.
https://doi.org/10.1186/s12966-023-01501-6 - Witte J, Foraita R, Didelez V. Multiple imputation and test-wise deletion for causal discovery with incomplete cohort data. Statistics in Medicine. 2022;41(23):4716-4743.
https://doi.org/10.1002/sim.9535 - Didelez V, Stensrud MJ. On the logic of collapsibility for causal effect measures. Biometrical Journal. 2022;64(2):235-242. (This paper has been recognized as a top cited and top downloaded research article from 2021-2022 in Biometrical Journal).
https://doi.org/10.1002/bimj.202000305 - Stensrud MJ, Young JG, Didelez V, Robins JM, Hernán MA. Separable effects for causal inference in the presence of competing events. Journal of the American Statistical Association. 2022;117(537):175-183.
https://doi.org/10.1080/01621459.2020.1765783 - Morris TT, Heron J, Sanderson E, Smith GD, Didelez V, Tilling K. Interpretation of Mendelian randomization using a single measure of an exposure that varies over time. International Journal of Epidemiology. 2022;51(6):1899-1909.
https://doi.org/10.1093/ije/dyac136 - Braitmaier M, Schwarz S, Kollhorst B, Senore C, Didelez V, Haug U. Screening colonoscopy similarly prevented distal and proximal colorectal cancer: A prospective study among 55-69-year-olds. Journal of Clinical Epidemiology. 2022;149:118-126.
https://doi.org/10.1016/j.jclinepi.2022.05.024 - Börnhorst C, Reinders T, Rathmann W, Bongaerts B, Haug U, Didelez V, Kollhorst B. Avoiding time-related biases: A feasibility study on antidiabetic drugs and pancreatic cancer applying the parametric g-formula to a large German healthcare database. Clinical Epidemiology. 2021;(13):1027-1038.
https://doi.org/10.2147/CLEP.S328342 - Andrews R, Didelez V. Insights into the cross-world independence assumption of causal mediation analysis. Epidemiology. 2021;32(2):209-219.
https://doi.org/10.1097/EDE.0000000000001313
https://repository.publisso.de/resource/frl%3A6425953 - Brydges CR, Carlson MC, Andrews R, Rebok GW, Bielak AAM. Using cognitive intraindividual variability to measure intervention effectiveness: Results from the Baltimore experience corps trial. The Journals of Gerontology. Series B, Psychological Sciences and Social Sciences. 2021;76(4):661-670.
https://doi.org/10.1093/geronb/gbaa009 - Stensrud MJ, Hernán MA, Tchetgen Tchetgen E, Robins JM, Didelez V, Young JG. A generalized theory of separable effects in competing event settings. Lifetime Data Analysis. 2021;27(4):588-631. (This paper was one of 2021's top downloaded Lifetime Data Analysis research articles).
https://doi.org/10.1007/s10985-021-09530-8 - Witte J, Henckel L, Maathuis MH, Didelez V. On efficient adjustment in causal graphs. Journal of Machine Learning Research. 2020;21(246):1-45.
http://jmlr.org/papers/v21/20-175.html - Andrews R, Shpitser I, Lopez O, Longstreth WT, Chaves PH, Kuller L, Carlson MC. Examining the causal mediating role of brain pathology on the relationship between diabetes and cognitive impairment: The Cardiovascular Health Study. Journal of the Royal Statistical Society. Series A (Statistics in Society). 2020;183(4):1705-1726.
https://doi.org/10.1111/rssa.12570 - Foraita R, Friemel J, Günther K, Behrens T, Bullerdiek J, Nimzyk R, Ahrens W, Didelez V. Causal discovery of gene regulation with incomplete data. Journal of the Royal Statistical Society. Series A (Statistics in Society). 2020;183(4):1747-1775.
https://doi.org/10.1111/rssa.12565 - Aalen OO, Stensrud MJ, Didelez V, Daniel R, Roysland K, Strohmaier S. Time-dependent mediators in survival analysis: Modeling direct and indirect effects with the additive hazards model. Biometrical Journal. 2020;62(3):532-549. (This paper has been recognized as a top cited research article from 2020-2021 in Biometrical Journal).
https://doi.org/10.1002/bimj.201800263
https://ui.adsabs.harvard.edu/abs/2020arXiv201113415A/abstract - Witte J, Didelez V. Covariate selection strategies for causal inference: Classification and comparison. Biometrical Journal. 2019;61(5):1270-1289.
https://doi.org/10.1002/bimj.201700294 - Didelez V. Defining causal mediation with a longitudinal mediator and a survival outcome. Lifetime Data Analysis. 2019;25(4):593-610. (This paper was one of 2020’s top downloaded and one of 2021's top cited Lifetime Data Analysis research articles).
https://doi.org/10.1007/s10985-018-9449-0 - Vansteelandt S, Didelez V. Improving the robustness and efficiency of covariate-adjusted linear instrumental variable estimators. Scandinavian Journal of Statistics, Theory and Applications. 2018;45(4):941-961.
https://doi.org/10.1111/sjos.12329
https://researchonline.lshtm.ac.uk/id/eprint/4658753/ - Farewell D, Huang C, Didelez V. Ignorability for general longitudinal data. Biometrika. 2017;104(2):317-326.
https://doi.org/10.1093/biomet/asx020 - Didelez V, Evans R. Causal inference from case-control studies. In: Borgan O, Breslow N, Chatterjee N, Gail M, Scott A, Wild C, Herausgeber. Handbook of statistical methods for case-control studies. Boca Raton: Chapman & Hall/CRC. 2018. S. 87-115.
- Didelez V. Causal concepts and graphical models. In: Maathuis M, Drton M, Lauritzen SL, Wainwright M, Herausgeber. Handbook of graphical models. Boston: CRC Press. 2018. S. 353-380.
- Didelez V. Causal estimands and interventions. "Learning from Interventions"-Workshop at the Simons Institute for the Theory of Computing, 14-17 February 2022, Berkeley, USA.
- Didelez V. Causal reasoning in survival and time-to-event analyses. Online Causal Inference Seminar, 1 December 2020, online presentation.
- Bang CW, Witte J, Foraita R, Didelez V. Efficient use of temporal background knowledge for causal discovery with cohort data. American Causal Inference Conference (ACIC), 23-25 May 2022, Berkeley, USA.
- Foraita R, Witte J, Börnhorst C, Pigeot I, Didelez V, on behalf of the I.Family and GrowH! consortia. A longitudinal causal graph analysis investigating modifiable risk factors and obesity in a European cohort of children and adolescents. 6. Konferenz der Deutschen Arbeitsgemeinschaft Statistik (DAGStat), 28. März-1. April 2022, Hamburg.
- Didelez V. Invited commentary: Where do the causal DAGs come from? American Journal of Epidemiology. 2024;193(8):1075-1078.
https://doi.org/10.1093/aje/kwae028 - Didelez V. Seconder of the vote of thanks to Vansteelandt and Dukes and contribution to the Discussion "Assumption-lean inference for generalized linear model parameters". Journal of the Royal Statistical Society. Series B (Statistical Methodology). 2022;84(3):689-691.
https://doi.org/10.1111/rssb.12514 - Fulcher I, Shpitser I, Didelez V, Zhou K, Scharfstein DO. Discussion on "Causal mediation of semicompeting risks" by Yen-Tsung Huang. Biometrics. 2021;77(4):1165-1169.
https://doi.org/10.1111/biom.13519
Conference Proceedings
Zeitschriftenartikel mit peer-review
Buchbeiträge
Vorträge bei wissenschaftlichen Tagungen (eingeladen)
Poster bei wissenschaftlichen Tagungen
Commentaries
Aktuelle Projekte
Es werden nur momentan laufende Projekte angezeigt, zu denen noch Veröffentlichungen erstellt werden oder solche, die vor weniger als einem Jahr endeten. Die Einträge sind alphabetisch sortiert.
- Der Intelligente Digitale Leitlinien-Editor (IDEAL): Anwendung kausaler Inferenz zur Evidenzgewinnung mittels emulierter klinischer Studien
- Graduiertenkolleg π³: Parameter Identifikation - Analyse, Agorithmen, Anwendungen
- Kausale Modellselektion für Kohortendaten
- KI-FOR Lifespan AI: Von longitudinalen Gesundheitsdaten zur Inferenz im Lebensverlauf
- Sonderforschungsbereich 1320: Wissenschaft der Alltagsaktivitäten - Analytische und generative Modellierung
Mitarbeiter:innen
Bergen, Luca
Tel.: +49 (0)421 218-56796
bergen(at)leibniz-bips.de
Börnhorst, Claudia, Dr. rer. nat.
Tel.: +49 (0)421 218-56946
Fax: +49 (0)421 218-56941
boern(at)leibniz-bips.de
Didelez, Vanessa, Prof. Dr. rer. nat.
Tel.: +49 (0)421 218-56939
Fax: +49 (0)421 218-56941
didelez(at)leibniz-bips.de
Frielinghaus, Maria
Tel.: +49 (0)421 218-56791
Frielinghaus(at)leibniz-bips.de
Runge, Lea
Tel.: +49 (0)421 218-56979
runge(at)leibniz-bips.de
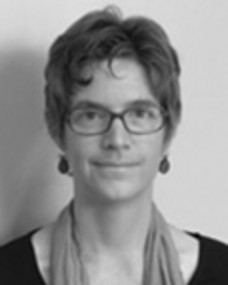
Vanessa Didelez
Stv. Abteilungsleiterin (Forschungsgruppenleiterin)
Kontakt:
Prof. Dr. rer. nat.
Vanessa Didelez
Tel: +49 (0)421 218-56939
Fax: +49 (0)421 218-56941